The US National Science Foundation National Center for Atmospheric Research (NSF NCAR) has released a platform, named the Community Research Earth Digital Intelligence Twin (CREDIT), which aims to lower the barriers to using artificial intelligence (AI) in weather research.
The platform has three parts: a library of AI models, multiple pre-prepared, high-quality datasets to train those models, and access to high-performance computing. This publicly available model is intended to enable researchers to choose between different model architectures and databases and then help them train the model without having extensive knowledge of high-performance computing.
“When our team started to build our first AI weather model, we found little publicly available guidance on how to train these models at full scale. We had to learn the hard way through trial and error and many discussions with other experts,” said David John Gagne, who leads NSF NCAR’s machine learning science efforts. “Our goal is to provide a platform that allows our research community to easily train models on particular datasets and then configure them to run on our available computing resources, all without having to be an expert in AI or supercomputing. We want to lower the barriers to entry and get this potentially transformative technology into the hands of many more interested researchers.”
First steps toward a new kind of community model
The organization’s rationale for the initiative is that the more people use these community models, the better they become because users contribute their own improvements and upgrades to the model code. NSF NCAR also highlighted that traditional community computer models have challenges, such as the massive increases in computational resources required to increase model resolution to study smaller-scale, localized weather phenomena. For example, doubling a model’s resolution (both horizontally and vertically) reportedly requires a 16-fold increase in computing power. The intense computational demand of these models also limits the number of times a model can be run.
The researchers also asserted that traditional models struggle in areas where scientific understanding of how a phenomenon forms or behaves is incomplete and therefore cannot be as readily expressed using mathematical equations. These tend to be the weather events that are stubbornly hard to accurately predict, such as the rapid intensification of hurricanes or the growth of damaging hail.
For these reasons, AI weather models are projected to be able to address these shortcomings. Unlike traditional models, AI models do not solve individual equations. Instead, they look for patterns and associations in the data that give insight into what’s likely to happen next. This approach significantly reduces computing demand and also means the models can find ways to predict phenomena for which equations cannot easily be written.
According to the organization, this potential and the rapid advance of AI have led to a profusion of new models developed by tech companies, nonprofits, government agencies and universities, which also have drawbacks, including a tendency for small errors to grow quickly into large ones. The NSF NCAR researchers pointed out that AI weather models are only as good as the data used to train them, and different questions may require using different training data, which can be difficult to source and prepare for use by AI models.
CREDIT is NSF NCAR’s first foray into building a community AI modeling platform to help facilitate progress in Earth system science research. The organization stated that there is still work to be done before CREDIT is a robust community modeling resource, and drew attention to the heavy reliance of its community models on thorough documentation, training and support infrastructure, which has not yet been built out for CREDIT.
“We want scientists to be able to focus on their research goals, rather than the technical details,” Gagne said. “This framework makes AI accessible to a broad range of users, from experienced atmospheric researchers to students just beginning their journeys.”
Competitive with traditional weather models
In the process of building and testing CREDIT, the NSF NCAR team also built WXFormer, a new AI weather model designed to be used for weather research questions and to address some of the shortfalls of existing AI weather modeling, including new strategies for limiting error growth and facilitating shorter time steps. Most existing AI weather models provide information in six-hour intervals, but WXFormer can provide forecasts hourly. WXFormer is now one of the models researchers can choose from within CREDIT’s library, along with others that have their codes published publicly.
Using CREDIT to train and set up the models, the NSF NCAR team tested the performance of WXFormer and FuXi, another AI model available in CREDIT’s library, against forecasts from the high-resolution integrated forecast system (HRES-IFS), a standard weather model developed by the European Centre for Medium-Range Weather Forecasts (ECMWF).
Both WXFormer and FuXi reportedly demonstrated they could provide the same or better forecasts than HRES-IFS at extended lead times for most atmospheric variables. For example, scientists tested how well the models predicted the track and intensity of Hurricane Laura, which struck western Louisiana as a Category 4 storm in 2020. They found that WXFormer accurately predicted the hurricane’s intensity at five days out, though its predicted track was too far south. A version of WXFormer that produces hourly forecasts captured the hurricane’s track more accurately but predicted a much weaker storm at five days out. The FuXi model predicted a weak storm at five days out and had similar track errors to WXFormer. The HRES-IFS model also predicted a very weak storm at five days out and had some track errors in the opposite direction of the AI models.
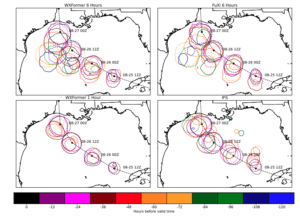
In all, the results underscore the potential of CREDIT and point to directions for future work. Already, the team has produced an updated release of CREDIT software that increases the platform’s user friendliness and scalability. They are also working on another AI model that can emulate the NSF NCAR-based Community Atmosphere Model (CAM), which is a component of the larger Community Earth System Model, which connects models of ocean, atmosphere, land and ice together. The ‘CAMulator’ would make it easier for AI modeling to be integrated into global Earth system simulations.
CREDIT’s development is being underpinned by a parallel effort at NSF NCAR to build an “integrated data commons” that would make the organization’s datasets more accessible for training AI models.
“We’re excited to see what problems CREDIT can help solve and where this can go with the help and participation of the broader community,” Gagne said. “Our aim is for CREDIT to be an open and collaborative environment where researchers at various levels of expertise can contribute to the framework’s evolution.”
In related news, the School of Atmospheric Sciences at Nanjing University, China, and the Earth System Numerical Prediction Center of the China Meteorological Administration recently published a paper, Impacts of lateral boundary conditions from numerical models and data-driven networks on convective-scale ensemble forecasts, in Atmospheric and Oceanic Science Letters, which outlines the impacts of the lateral boundary conditions provided by the AI models ‘Pangu-weather’ and ‘Fuxi-weather’ on convective-scale ensemble prediction systems. Click here to read the full story.